Earthquakes are a major concern in increasingly populated regions, however their prediction is a difficult task. Researchers have recently made progress in the use of complex simulation and modeling techniques to better forecast the occurrences of earthquakes.
In a recent study, researchers used Gradient Boosted Regression Trees, a machine learning technique for regression and classification problems that incorporates training data, to better determine spatiotemporally complex loading histories within subduction zones. The researchers simulated tens of earthquakes using a small‐scale experimental replica of a subduction zone and show that machine learning predicts well the timing and size of laboratory earthquakes by reconstructing and properly interpreting the spatiotemporally complex loading history of the system. These results promise substantial progress in real earthquake forecasting as they suggest that the complex motion recorded by geodesists at subduction zones might be diagnostic of earthquake imminence.
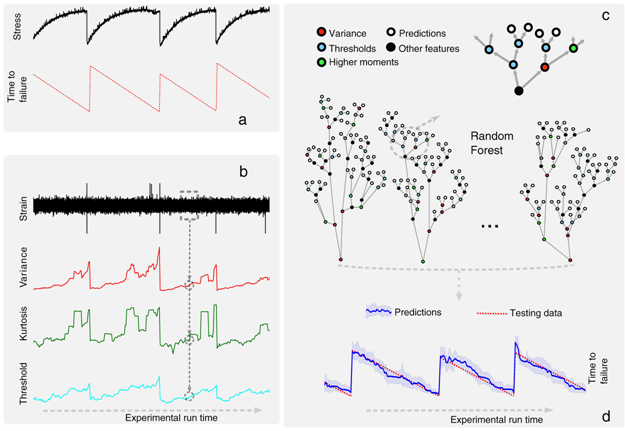
Other improvements have been also applied to early warning systems. Signals along the ocean can sometimes be deceptive as to the occurrence of earthquakes, sending false alarms regarding tsunamis and tectonic events. To mitigate this problem, a team of researchers recently used machine learning tools to determine if the waveforms are generated by earthquakes or local noise sources. They trained the algorithms with about 700,000 P-waves emitted by past earthquakes in southern California and Japan to better recognize the energy wave and differentiate it from other waves that may trigger a false alarm. Effectively, this better distinguishes what an earthquake-related wave is: in the study the accuracy was over 99% as to what waves constituted those related to earthquakes.